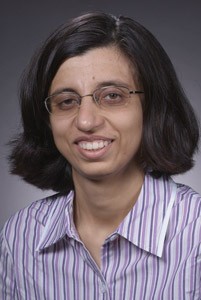
Namrata Vaswani
Email: namrata@iastate.edu
Phone: 515-294-4012
Title(s):
Endowed Anderlik Professor
Office
3121 Coover2520 Osborn Dr.
Ames, IA 500111046
Information
Education
Ph.D., Electrical and Computer Engineering, University of Maryland (2004)
B.Tech., Electrical Engineering, Indian Institute of Technology (1999)
Research Areas
Core Area(s): Communications and signal processing, statistical and sequential signal processing, recursive sparse reconstruction and compressive sensing, medical imaging
Department’s Strategic Area(s): Data, decisions, networks & autonomy; bioengineering
Publications
Google Scholar Profile: https://scholar.google.com/citations?user=s-dQPO8AAAAJ&hl=en
- Nayer, N. Vaswani, “Fast and sample-efficient federated low rank matrix recovery from column-wise linear and quadratic projections”, IEEE Trans. Information Theory, 2023
- Babu, S. Lingala, N. Vaswani, “Fast Low Rank Column-wise Compressive Sensing for Accelerated Dynamic MRI”,IEEE Trans. Computational Imaging, 2023
- Nayer*, N. Vaswani, “Sample-Efficient Low Rank Phase Retrieval”, IEEE Trans. Information Theory, Dec., 2021.
- Das, A. Ramamoorthy, N. Vaswani, “Efficient and Robust Distributed Matrix Computations via Convolutional Coding”, IEEE Trans. Information Theory, Sept., 2021.
- Narayanamurthy*, N. Vaswani, “Fast Robust Subspace Tracking via PCA in Sparse Data-Dependent Noise”, IEEE Journal on Selected Topics in Information Theory (JSAIT), special issue on Estimation and Inference, Nov. 2020
- Narayanamurthy*, N. Vaswani, “Provable Dynamic Robust PCA or Robust Subspace Tracking”, IEEE Trans. Information Theory, to appear, 2018.
- Narayanamurthy*, N. Vaswani, “Nearly Optimal Robust Subspace Tracking and Dynamic Robust PCA”, Proc. Intl. Conf. Machine Learning (ICML), 2018.
- Vaswani and P. Narayanamurthy*, “Static and Dynamic Robust Principal Component Analysis (PCA) and Matrix Completion: A Review”, Proceedings of the IEEE, August 2018 (special issue on Rethinking PCA for Modern Datasets).
- Vaswani and H. Guo*, “Correlated-PCA: Principal Components’ Analysis when Data and Noise are Correlated”, Proc. Neural Info. Proc. Systems (NIPS), 2016.
- Qiu*, N. Vaswani, B. Lois* and L. Hogben, “Recursive Robust PCA or Recursive Sparse Recovery in Large but Structured Noise”, IEEE Trans. Information Theory, August, 2014.