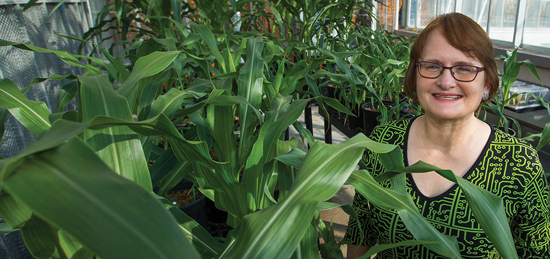
A computer network and a plant don’t seem to have much in common at first glance. But when Julie Dickerson looks, she sees many similarities – and sees how her expertise in computing network science can help unravel complex biological systems.
“Mapping networks of how genes influence plant growth and development is key to increasing crop productivity to help feed a growing world population,” says Dickerson, Northrop Grumman Professor in honor of Fred W. O’Green in electrical and computer engineering. “Bringing what we know about computing networks provides some methods to really examine the complicated genetic relationships.”
However, using the data science we know works in human-built systems becomes more challenging when mother nature gets involved.
“Computer systems were designed for simplicity and efficiency, but nature comes up with things humans could have never thought of,” says Dickerson. “Biological systems are really robust with a lot of redundancies, parallel pathways and even alternative pathways that lie dormant until just the right conditions are switched on.”
The combination of increasingly large amounts of available genetic data and the simple “we don’t know what we don’t yet know” about biological systems makes network modeling challenging.
Dickerson is focusing on alternative genetic splicing in plants, or the process of gene expression where one gene code can yield multiple proteins. First observed several decades ago, alternative splicing was thought to be the exception to the gene expression rule, but new genome sequencing technologies have revealed alternative splicing is very common. And very complex.
“We keep developing better tests and sensors that gather detailed data about gene expression, and all of a sudden we can see things going on with the genome that we didn’t know about before,” said Dickerson. “We now have so much more information to build network models with – that’s a challenge on its own. But we also know much mystery still remains, and we must model with that uncertainty – that’s the really interesting challenge.”
Dickerson and her team use machine learning to analyze huge genetic system data sets to make hypotheses about the flow of connections in gene expression. She also works closely with biologists who are experts in plant systems to help integrate their expertise into network models.
The ultimate goal is revealing the overarching governing principles of plants’ genetic expression.
“Data and computing capability are both increasing, and as time resolution improves, we will be able to really see what’s going on with genes and regulation” said Dickerson. “Understanding the network of gene expression is the first step to developing precise predictive breeding to improve plants’ health and productivity.”
Biologists + Engineers = New Approaches, New Solutions
Julie Dickerson is the principal investigator on a National Science Foundation Research Traineeship grant that brings together plant sciences, statistics and engineering to provide innovative training in a program called Predictive Plant Phenomics (P3).
“At Iowa State, we’ve done an excellent job marrying two of our longtime strengths: engineering and life sciences,” said Dickerson. “This work in data-driven plant science is only possible because of the unique combination of expertise.”
Co-principal investigators on the P3 NRT are Ted Heindel, Bergles Professor in Thermal Science in the Department of Mechanical Engineering; Carolyn Lawrence-Dill, associate professor of genetics, development and cell biology; and Pat Schnable, C.F. Curtiss Distinguished Professor in the Department of Agronomy and director of Iowa State’s Plant Sciences Institute.
The program will train 28 graduate students to effectively integrate diverse data streams and approaches, including plant data collection and analysis, sensor development, high-throughput robot technology, and plant image analysis.
“Leading faculty from departments collaborate on our NRT,” said Dickerson. “Our students are getting a one-of-a-kind education that will allow them to develop data-driven plant breeding and management improvements.”